
By
Primusvidya
Date
05/03/2024
AI V/S Machine Learning Differences & Advantages
Artificial intelligence (AI) and machine learning (ML) have become two of the most discussed emerging technologies in recent years. Though sometimes conflated as the same, they are distinct disciplines with their unique features and applications.
Artificial intelligence (AI) and machine learning (ML) have become two of the most discussed emerging technologies in recent years. Though sometimes conflated as the same, they are distinct disciplines with their unique features and applications.
However, both AI and ML offer tangible benefits for businesses. As Harvard Business Review says, Machine Learning (ML) now fundamentally elevates customer experiences - from personalized recommendations to highly relevant search results. Meanwhile, McKinsey anticipates over 75% of the value unleashed by generative Artificial Intelligence (AI) will transform customer operations, marketing, sales, engineering, and R&D.
Before delving into the countless ways companies leverage AI and ML to gain competitive advantages and craft standout customer experiences, it is instructive first to comprehend the difference between the two technologies and how they work in tandem to drive business innovation and transformation. This understanding sets the stage for more informed strategies for successfully deploying AI and ML across the enterprise.
What is Artificial Intelligence (AI)?
Artificial intelligence (AI) is the use of sophisticated analytical and logic-based techniques - such as machine learning - to interpret data, bolster and computerize decision-making, and enable technology systems to take autonomous actions. In essence, AI employs advanced algorithms to carry out tasks that would otherwise require human intelligence.
Artificial intelligence Applications
Artificial intelligence refers to the field of computer science focused on developing systems that can mimic human-level intelligence and perform tasks typically requiring human cognition. The core goal of AI is to enable automation by creating machines that can function and react similarly to the human mind - adapting to diverse situations and progressively enhancing their capabilities through accumulated experience.
AI can be categorized into two primary types - Narrow or Weak AI, and General or Strong AI. Narrow AI refers to systems designed for specialized, well-defined tasks such as virtual assistants, self-driving vehicles, and facial recognition technology. These exhibit narrowly focused and constrained intelligence. General AI hypothesizes machines with generalized, human-range intelligence and versatility to accomplish any intellectual task at human capability levels or beyond. While narrow AI has seen growing practical applications, general human-level AI remains theoretical. Current AI mostly aims to augment, not replicate the nuances and breadth of human cognition.
What is Machine Learning (ML)?
Pioneering artificial intelligence scientist Arthur Samuel coined the term "machine learning" in the 1950s, defining it as the domain focused on empowering computers to gain knowledge and improve performance without needing explicit programming for every scenario.
In contemporary times, machine learning has become deeply embedded in multitudes of common digital interactions - from using messaging predictive text and online recommendations to engaging with conversational chatbots. Through techniques like predictive analytics, machine learning allows these systems and platforms to evolve based on interpretations of behavioral data and previous choices rather than depending solely on predefined logic structures. Essentially, the algorithms "learn" patterns and adapt accordingly instead of requiring human input to manually code all variations. The niche yet expanding presence of machine learning today likely signals more mainstream integration across information systems going forward.
How Does Machine Learning Work?
Machine learning and artificial intelligence are distinct yet deeply interdependent concepts. Machine learning forms the backbone for many AI applications by providing the mechanisms for adaptation and improvement. In other words, machine learning allows AI systems to evolve based on learned experiences rather than requiring explicit programming for every possible scenario.
Consider Siri, a well-known virtual assistant. Its capacity to grasp natural language and conversational nuances reflects the AI aspect. Meanwhile, machine learning enables Siri to incrementally boost its speech recognition accuracies based on user interactions over time, rather than solely relying on predefined programming.
In summary, while artificial intelligence broadly focuses on creating systems that operate with human-level intelligence, machine learning offers specific tools and algorithms to realize that goal. It empowers AI solutions to independently augment their decision-making and performances through continual learning. Machine learning delivers the means; artificial intelligence defines the ends. Working in unity, they enable technology that keeps pace with dynamic real-world environments.
Large Language Models Used to Train AI
A large language model (LLM) leverages deep learning and vast data sets to algorithmically understand, summarize, generate, and predict textual content.
In recent times, advances in large language models have emerged as a breakthrough in generative artificial intelligence - with capacities to process and produce substantial volumes of coherent writing. Models such as OpenAI's ChatGPT have been trained on massive text corpora to acquire skills including translation, answering questions, automating content creation and even coding.
The architecture underpinning these models comprises neural networks with successive layers of connected nodes that iteratively extract insights from input data. Via a training process that continuously refines internal parameters, the model learns to minimize the divergence between its textual outputs and the patterns in the source training data. In effect, large language models employ scale and computational pattern recognition to absorb the nuances of human languages and communication. Their flexible generative abilities hold promise to transform industries involving written content.
How Companies are using AI and ML
Practical applications of artificial intelligence (AI) and machine learning (ML) technologies are proliferating rapidly across sectors. As innovations continue advancing AI and ML capabilities, both agile startups and industry stalwarts are harnessing them to tackle intricate business challenges, optimize processes, and unlock new sources of value. From personalized marketing to predictive analytics, some of the most widespread deployments of AI and ML that are driving this pervasive digital transformation include:
- Data analysis and insights: Machine learning models are leveraged to examine substantial data corpora and pinpoint actionable patterns and intelligence. By empowering businesses to interpret volumes of data more efficiently than manual analysis permits, machine learning drives enhanced decision-making, forecasting, and opportunity recognition powered by data-derived insights rather than intuition alone. The scalable capacity of machine learning algorithms to process data and spotlight meaningful interrelationships supports organizations across functions - from sales to risk to marketing and beyond - to base strategic choices on evidence-led insights for superior outcomes.
- Customer Experience Enhancement: Artificial intelligence-enabled chatbots and virtual assistants are gaining traction across companies seeking to boost customer experience. These AI agents offer real-time service by engaging users in natural conversations to understand support needs, provide customized solutions, and rapidly resolve requests.1728 Unlike traditional support models that follow rigid protocols, AI-powered assistants can dynamically adapt interactions based on user context and history to emulate empathetic human-like responses. The scalability also facilitates concurrent assistance for multitudes of customers. With continual learning and empathy-focused design, AI chatbots are enabling businesses to deliver flexible yet personalized customer journeys - interacting intelligently to enhance satisfaction while increasing operational efficiency.
- Healthcare Diagnostics: Artificial intelligence is increasingly uplifting medical imaging diagnoses through its adeptness in discerning hard-to-perceive visual patterns. Machine learning models can be trained to meticulously analyze medical scans with granularity beyond human capacity. These algorithms systematically mine datasets of images to identify signatures associated with various diseases based on textural patterns imperceptible to the naked eye. Doctors are now utilizing such AI tools to evaluate scans and uncover early traces of conditions like cancer with enhanced accuracy and speed. By scaling specialized analysis to augment clinical workflows, artificial intelligence promises to boost productivity while attaining new diagnostics precision levels to improve patient outcomes. Its growing integration reflects AI’s rising value in unlocking latent insights within medical visuals to advance life-critical evaluation and decision-making.
- Autonomous Vehicles: Machine learning forms an integral component powering robotic automation and autonomous vehicle platforms. Self-navigating transports like driverless cars employ a symphony of technologies - from computer vision to continuously interpret environmental stimuli to machine learning for enhancing decision-making abilities based on accrued experiences. Unlike conventional vehicles, autonomous cars utilize real-time sensory data and machine learning-activated neural networks to perpetually optimize navigation and make split-second maneuvering judgments that maximize passenger safety. By enabling systems to independently extract insights from live data flow to improve responses, machine learning institutes another layer of dynamic intelligence to replace static programming. This allows emerging smart machines to dynamically adapt to fluid real-world conditions in a human-like fashion - a flexibility crucial for safety across application domains.
- Financial Services: Artificial intelligence systems are increasingly leveraged for real-time fraud detection based on their proficiency in identifying aberrant transaction motifs instantly. AI fraud solutions employ algorithms trained to intricately analyze streams of transaction data and discern activity diverging from established patterns that may suggest illegal behavior. By automatically flagging anomalies as they emerge amidst volumes of interactions, AI enables preemptive fraud identification in a proactive capacity unmatched by manual means. Such real-time detection facilitates intervention before incidents escalate in scale and severity. Going forward, the analytics responsiveness furnished by AI fraud systems promises to mitigate financial risks by acting as perpetual vigilance layers attuned to the slightest indicators of suspicious conduct through data-driven intelligence.
- Manufacturing and supply chain optimization: Artificial intelligence is transforming manufacturing plant maintenance from reactive to prescriptive practices. AI predictive engines analyze sensor data across equipment to identify early performance deviations and nascent failure signatures. By perpetual monitoring machine conditions against baseline health metrics, aberrant sensor readings can trigger predictive alerts to imminent breakdown risks – well before outages manifest. This foresight enables just-in-time maintenance interventions rather than routine servicing. Consequently, this prescriptive approach powered by predictive AI minimizes downtimes and maximizes manufacturing continuity. As AI models grow more attuned to the operating rhythms of complex machinery, they promise to slash maintenance overheads through ultra-precise failure forecasts optimized for each asset’s aging patterns and usage profiles.
- Content Recommendations: ML is utilized by online content providers, streaming platforms, and e-commerce companies to examine user preferences and behavior, thereby offering tailored content suggestions to enhance user interaction. Through the analysis of unstructured data, marketers can further comprehend which content and keywords to persist with.
- Cybersecurity: AI and ML algorithms play a crucial role in analyzing network traffic, URLs, and DNS requests, enabling the identification and prevention of malicious websites, phishing attempts, malware downloads, and various cyber threats.
Artificial Intelligence and Machine Learning: Shaping the Future Ahead
Artificial Intelligence (AI) and Machine Learning (ML) synergize to revolutionize various sectors, from marketing to healthcare. They optimize experiences, offering personalized recommendations, predictive maintenance, and efficient operations. As AI and ML advance, industries witness transformative shifts, enhancing efficiency and innovation. In marketing, targeted ads increase engagement; in healthcare, AI aids diagnostics and treatment plans. These technologies redefine conventional practices, propelling industries into a future characterized by unparalleled growth and efficacy.
Recent Blogs

A Diploma in Education (D.Ed) is a professional qualificatio...
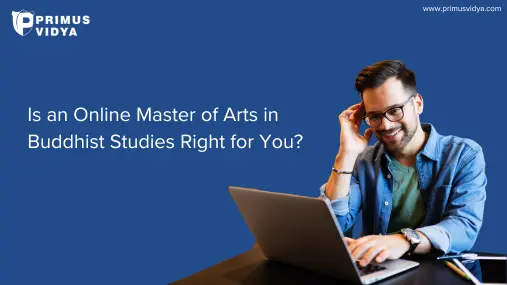
The Online Master of Arts (Online MA) in Buddhist Studies is...

Work Integrated Learning Programs (WILP) are designed for wo...

In the dynamic and ever-evolving domain of education, the re...

Initializing a career in teaching within Uttar Pradesh requi...
Have a Query?
Fill out the form below & we will get back to you.
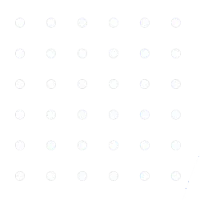